Hima Lakkaraju
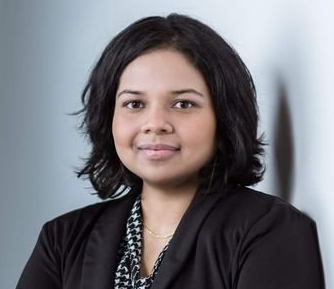
Contact
hlakkaraju@hbs.eduhlakkaraju@seas.harvard.edu
Morgan Hall 491
Science and Engineering Complex 6.220
@hima_lakkaraju
lvhimabindu
I am an Assistant Professor at Harvard University with appointments in the Business School and the Department of Computer Science. Prior to my stint at Harvard, I received my PhD in Computer Science from Stanford University.
My research interests lie within the broad area of the algorithmic foundations and societal implications of trustworthy AI. Specifically, I develop machine learning and optimization techniques as well as evaluation frameworks to improve the safety, interpretability, fairness, privacy, and reasoning capabilities of predictive and generative models, including large language models (LLMs). My work addresses fundamental questions at the intersection of human and algorithmic decision-making, such as:
- How can we build interpretable and accurate models to assist in human decision-making?
- How do we identify and correct underlying biases in both human decisions and model predictions?
- How can we ensure that models and their interpretations are robust to adversarial and privacy attacks?
- How do we train and evaluate models in the face of missing counterfactuals and unmeasured confounding?
These questions have far-reaching implications in domains involving high-stakes decisions such as health care, policy, law, and business.
I lead the AI4LIFE research group at Harvard and I recently co-founded the Trustworthy ML Initiative (TrustML) to help lower entry barriers into trustworthy ML and bring together researchers and practitioners working in the field. My research is being generously supported by NSF, Sloan Foundation, Schmidt Sciences, Google, OpenAI, Amazon, JP Morgan, Adobe, Bayer, Harvard Data Science Initiative, and D^3 Insitute at Harvard. My work has been featured in various major media outlets including the New York Times, TIME magazine, Fortune, Forbes, MIT Technology Review, and Harvard Business Review.
Please check out my CV for more details about me and my research.
NOTE: I am looking for motivated graduate and undergraduate students and postdocs who are broadly interested in trustworthy machine learning and large pre-trained models. If you are excited about this line of research and would like to work with me, please read this before contacting me.
Selected Achievements & News
- Alfred P. Sloan Fellow in Computer Science, 2025
- Outstanding Paper Award at New England NLP Symposium, 2025
- Excited to serve as the ethics co-chair (again!) for NeurIPS 2025
- Distinguished Young Alumnus Award, Indian Institute of Science, 2024
- Excited to serve as the ethics co-chair for NeurIPS 2024
- AI2050 Early Career Fellowship by Schmidt Sciences, 2024
- NSF CAREER Award, 2023
- Named Kavli Fellow 2023 by National Academy of Sciences
- Adobe Data Science Research Award, 2023
- Excited to co-organize the first workshop on Regulatable ML: Bridging the Gaps between ML Research and Regulations at NeurIPS, 2023.
- Excited to serve as the tutorial co-chair for WSDM 2024
- Excited to serve as the sponsorship co-chair for FAccT 2023
- My short course on Explainable AI hosted by Stanford University is now available on Youtube (over 90,000 views)
- Outstanding Paper Award Honorable Mention, NeurIPS Workshop on Trustworthy and Socially Responsible Machine Learning, 2022
- JP Morgan Faculty Research Award, 2022
- Best Paper Award, ICML Workshop on Interpretable ML in Healthcare, 2022
- Released the first version of OpenXAI, a light-weight open source library to evaluate and benchmark post hoc explanation methods, 2022
- Amazon Research Award, 2021
- Google AI for Social Good Research Award, 2021
- Best Paper Runner Up, ICML Workshop on Algorithmic Recourse, 2021
- Google Research Award, 2020
- Hoopes prize for undergraduate thesis mentoring, Harvard University, 2020
- Co-founded Trustworthy ML Initiative to enable easy access to resources on trustworthy ML & to build a community of researchers/practitioners, 2020
- Named one of the world's 35 innovators under 35 by MIT Tech Review, 2019
- Named one of the world's top innovators to watch by Vanity Fair, 2019
- Selected for the prestigious Cowles fellowship by Yale University, 2018
- INFORMS Best Data Mining Paper Award, 2017
- Microsoft Research Dissertation Grant, 2017
- Named a Rising Star in Computer Science, 2016
- Google Anita Borg Fellowship , 2015
- Stanford Graduate Fellowship, 2013-17
- Eminence and Excellence Award, IBM Research, 2012
- Research Division Award, IBM Research, 2012
- Best Paper Award, SIAM International Conference on Data Mining, 2011
Upcoming and Recent Talks
11.2024 EMNLP Workshop on BlackboxNLP10.2024 Learning Machines Seminar, Cornell University10.2024 First Annual Summit on Responsible Computing, AI, and Society, Georgia Tech10.2024 AI Alignment Workshop, University of Maryland09.2024 Workshops on AI Regulation, India and Singapore04.2024 Princeton University Workshop on Understanding in Natural and Artificial Minds04.2024 Johns Hopkins CS Seminar Series-
03.2024 US Securities and Exchange Commission 03.2024 Guest Lecture in Algorithmic Fairness Course, Cornell University02.2024 MIT Data Science Seminar Series02.2024 UPenn Center for Safe, Explainable, and Trustworthy AI Seminar Series02.2024 AAAI Workshop on Privacy-Preserving Artificial Intelligence-
01.2024 NSF Workshop on Advanced Automated Systems, Contestability, and the Law -
10.2023 Yale and Google Joint Workshop on Theory and Practice of Foundation Models -
10.2023 Google, Stanford, and UW Madison Workshop on Securing the Future of GenAI -
10.2023 INFORMS Annual Meeting -
07.2023 ICML Workshop on Interpretable ML in Healthcare -
07.2023 ICML Workshop on Counterfactuals in Minds and Machines -
07.2023 RSS Workshop on Safe Autonomy -
05.2023 ICLR Workshop on Trustworthy and Reliable Large-Scale Machine Learning Models -
05.2023 Responsible AI Workshop at Carnegie Mellon University -
04.2023 Guest Lecture at Carnegie Mellon University -
04.2023 Mind and Machine Intelligence Summit, UC Santa Barbara -
04.2023 ACM India Bootcamp on Responsible Computing -
03.2023 Cornell University and Weill Cornell Medicine -
03.2023 Guest Lecture at UC Berkeley -
03.2023 Kavli Frontiers of Science Symposium -
03.2023 Cohere AI -
02.2023 Keynote at AAAI Workshop on Representation Learning for Responsible Human-Centric AI -
02.2023 AAAI Workshop on Deployable AI -
12.2022 Berkman Klein Center, Harvard University -
11.2022 Keynote at Women in Machine Learning (WiML) Workshop Co-located with NeurIPS, 2022 -
11.2022 Machine Learning for Health (ML4H) Workshop Co-located with NeurIPS, 2022 -
11.2022 Simons Institute (Berkeley) Workshop on Societal Considerations and Applications -
11.2022 MIT Initiative on the Digital Economy (IDE) Seminar Series -
11.2022 Harvard Data Science Initiative's Annual Conference -
10.2022 ECCV Workshop on Adversarial Robustness in the Real World -
08.2022 Stanford Center for AI Safety -
08.2022 Amazon Alexa Rising Star Speaker Series -
06.2022 CVPR Workshop on Explainable AI for Computer Vision -
05.2022 ICLR Workshop on Privacy, Accountability, Interpretability, Robustness, Reasoning on Structured Data -
05.2022 Keynote at WWW Workshop on Explainable AI in Health -
05.2022 Fiddler AI Fireside Chat -
04.2022 AI and the Economy (U.S. Department of Commerce, National Institute of Standards and Technology, Stanford HAI, and the FinRegLab) -
04.2022 Stanford Human-Centered Artificial Intelligence (HAI) Conference -
04.2022 Stanford Digital Econ Seminar -
03.2022 University of Southern California
Research
- * below indicates equal contribution
Selected Preprints
- See Google Scholar Page for a full list of preprints
-
How Post-Training Reshapes LLMs: A Mechanistic View on Knowledge, Truthfulness, Refusal, and Confidence
Hongzhe Du, Weikai Li, Min Cai, Karim Saraipour, Zimin Zhang, Himabindu Lakkaraju, Yizhou Sun, Shichang Zhang
Outstanding Paper Award, New England NLP Symposium 2025
pdf -
Building Bridges, Not Walls -- Advancing Interpretability by Unifying Feature, Data, and Model Component Attribution
Shichang Zhang, Tessa Han, Usha Bhalla, Himabindu Lakkaraju
pdf -
Operationalizing the Blueprint for an AI Bill of Rights: Recommendations for Practitioners, Researchers, and Policy Makers
Alex Oesterling, Usha Bhalla, Suresh Venkatasubramanian, Himabindu Lakkaraju
pdf -
Generalized Group Data Attribution
Dan Ley, Suraj Srinivas, Shichang Zhang, Gili Rusak, Himabindu Lakkaraju
pdf -
Generalizing Trust: Weak-to-Strong Trustworthiness in Language Models
Martin Pawelczyk, Lillian Sun, Zhenting Qi, Aounon Kumar, Himabindu Lakkaraju
pdf -
On the Hardness of Faithful Chain-of-Thought Reasoning in Large Language Models
Sree Harsha Tanneru, Dan Ley, Chirag Agarwal, Himabindu Lakkaraju
Research Highlight: OpenAI o1 System Card
pdf -
Manipulating Large Language Models to Increase Product and Content Visibility
Aounon Kumar, Himabindu Lakkaraju
Press: The New York Times | The Guardian | Communications of the ACM | Towards Data Science
pdf
Publications
-
On the Impact of Fine-Tuning on Chain-of-Thought Reasoning
Elita Lobo, Chirag Agarwal, Himabindu Lakkaraju
The North American Chapter of the Association for Computational Linguistics (NAACL), 2025.
pdf -
Quantifying Generalization Complexity for Large Language Models
Zhenting Qi, Hongyin Luo, Xuliang Huang, Zhuokai Zhao, Yibo Jiang, Xiangjun Fan, Himabindu Lakkaraju, James Glass
International Conference on Learning Representations (ICLR), 2025.
pdf -
More RLHF, More Trust? On The Impact of Preference Alignment On Trustworthiness
Aaron Jiaxun Li, Satyapriya Krishna, Himabindu Lakkaraju
International Conference on Learning Representations (ICLR), 2025.
Oral Presentation [Top 1.8%]
pdf -
Follow My Instruction and Spill the Beans: Scalable Data Extraction from Retrieval-Augmented Generation Systems
Zhenting Qi, Hanlin Zhang, Eric P. Xing, Sham M. Kakade, Himabindu Lakkaraju
International Conference on Learning Representations (ICLR), 2025.
pdf -
Interpreting CLIP with Sparse Linear Concept Embeddings (SpLiCE)
Usha Bhalla, Alex Oesterling, Suraj Srinivas, Flavio Calmon, Himabindu Lakkaraju
Advances in Neural Information Processing Systems (NeurIPS), 2024.
pdf -
MedSafetyBench: Evaluating and Improving the Medical Safety of Large Language Models
Tessa Han, Aounon Kumar, Chirag Agarwal, Himabindu Lakkaraju
Advances in Neural Information Processing Systems (NeurIPS), 2024.
pdf -
In-context Unlearning: Language Models as Few Shot Unlearners
Martin Pawelczyk, Seth Neel, Himabindu Lakkaraju
International Conference on Machine Learning (ICML), 2024.
pdf -
Understanding the Effects of Iterative Prompting on Truthfulness
Satyapriya Krishna, Chirag Agarwal, Himabindu Lakkaraju
International Conference on Machine Learning (ICML), 2024.
pdf -
Characterizing Data Point Vulnerability as Average-Case Robustness
Tessa Han, Suraj Srinivas, Himabindu Lakkaraju
International Conference on Uncertainty in Artificial Intelligence (UAI), 2024.
pdf -
A Study on the Calibration of In-context Learning
Hanlin Zhang, Yi-Fan Zhang, Yaodong Yu, Dhruv Madeka, Dean Foster, Eric Xing, Himabindu Lakkaraju, Sham Kakade
The North American Chapter of the Association for Computational Linguistics (NAACL), 2024.
pdf -
Investigating the Fairness of Large Language Models for Predictions on Tabular Data
Yanchen Liu, Srishti Gautam, Jiaqi Ma, Himabindu Lakkaraju
The North American Chapter of the Association for Computational Linguistics (NAACL), 2024.
pdf -
Quantifying Uncertainty in Natural Language Explanations of Large Language Models
Sree Harsha Tanneru, Chirag Agarwal, Himabindu Lakkaraju
International Conference on Artificial Intelligence and Statistics (AISTATS), 2024.
Spotlight Presentation, NeurIPS Workshop on Robustness of Few-shot and Zero-shot Learning in Foundation Models, 2023.
pdf -
Fair Machine Unlearning: Data Removal while Mitigating Disparities
Alex Oesterling, Jiaqi Ma, Flavio Calmon, Himabindu Lakkaraju
International Conference on Artificial Intelligence and Statistics (AISTATS), 2024.
pdf -
The Disagreement Problem in Explainable Machine Learning: A Practitioner's Perspective
Satyapriya Krishna*, Tessa Han*, Alex Gu, Javin Pombra, Shahin Jabbari, Steven Wu, Himabindu Lakkaraju
Transactions on Machine Learning Research (TMLR), 2024.
Press: Fortune Magazine
pdf -
Certifying LLM Safety Against Adversarial Prompting
Aounon Kumar, Chirag Agarwal, Suraj Srinivas, Aaron Li, Soheil Feizi, Himabindu Lakkaraju
Conference on Language Modeling (COLM), 2024.
pdf -
TalkToModel: Explaining Machine Learning Models with Interactive Natural Language Conversations
Dylan Slack, Satyapriya Krishna, Himabindu Lakkaraju*, Sameer Singh*
Nature Machine Intelligence, 2023.
Outstanding Paper Award Honorable Mention, NeurIPS Workshop on Trustworthy and Socially Responsible ML, 2022.
pdf -
Evaluating Explainability for Graph Neural Networks
Chirag Agarwal, Owen Queen, Himabindu Lakkaraju, Marinka Zitnik
Nature Scientific Data, 2023.
pdf -
Post Hoc Explanations of Language Models Can Improve Language Models
Satyapriya Krishna, Jiaqi Ma, Dylan Slack, Asma Ghandeharioun, Sameer Singh, Himabindu Lakkaraju
Advances in Neural Information Processing Systems (NeurIPS), 2023.
pdf -
Verifiable Feature Attributions: A Bridge between Post Hoc Explainability and Inherent Interpretability
Usha Bhalla*, Suraj Srinivas*, Himabindu Lakkaraju
Advances in Neural Information Processing Systems (NeurIPS), 2023.
pdf -
Which Models have Perceptually-Aligned Gradients? An Explanation via Off-Manifold Robustness
Suraj Srinivas*, Sebastian Bordt*, Himabindu Lakkaraju
Advances in Neural Information Processing Systems (NeurIPS), 2023.
Spotlight Presentation [Top 3%]
pdf -
M4: A Unified XAI Benchmark for Faithfulness Evaluation of Feature Attribution Methods across Metrics, Modalities, and Models
Xuhong Li, Mengnan Du, Jiamin Chen, Yekun Chai, Himabindu Lakkaraju, Haoyi Xiong
Advances in Neural Information Processing Systems (NeurIPS), 2023.
-
When Does Uncertainty Matter?: Understanding the Impact of Predictive Uncertainty in ML Assisted Decision Making
Sean McGrath, Parth Mehta, Alexandra Zytek, Isaac Lage, Himabindu Lakkaraju
Transactions on Machine Learning Research (TMLR), 2023.
pdf -
Towards Bridging the Gaps between the Right to Explanation and the Right to be Forgotten
Satyapriya Krishna*, Jiaqi Ma*, Himabindu Lakkaraju
International Conference on Machine Learning (ICML), 2023
pdf -
On the Impact of Actionable Explanations on Social Segregation
Ruijiang Gao, Himabindu Lakkaraju
International Conference on Machine Learning (ICML), 2023
pdf -
On Minimizing the Impact of Dataset Shifts on Actionable Explanations
Anna Meyer*, Dan Ley*, Suraj Srinivas, Himabindu Lakkaraju
Conference on Uncertainty in Artificial Intelligence (UAI), 2023
Oral Presentation [Top 5%]
pdf -
Probabilistically Robust Recourse: Navigating the Trade-offs between Costs and Robustness in Algorithmic Recourse
Martin Pawelczyk, Teresa Datta, Johannes van-den-Heuvel, Gjergji Kasneci, Himabindu Lakkaraju
International Conference on Learning Representations (ICLR), 2023
pdf -
On the Privacy Risks of Algorithmic Recourse
Martin Pawelczyk, Himabindu Lakkaraju, Seth Neel
International Conference on Artificial Intelligence and Statistics (AISTATS), 2023
pdf -
Which Explanation Should I Choose? A Function Approximation Perspective to Characterizing Post hoc Explanations
Tessa Han, Suraj Srinivas, Himabindu Lakkaraju
Advances in Neural Information Processing Systems (NeurIPS), 2022.
Best Paper Award, ICML Workshop on Interpretable Machine Learning in Healthcare, 2022.
pdf -
Flatten the Curve: Efficiently Training Low-Curvature Neural Networks
Suraj Srinivas, Kyle Matoba, Himabindu Lakkaraju, Francois Fleuret
Advances in Neural Information Processing Systems (NeurIPS), 2022.
pdf -
OpenXAI: Towards a Transparent Evaluation of Model Explanations
Chirag Agarwal, Satyapriya Krishna, Eshika Saxena, Martin Pawelczyk, Nari Johnson, Isha Puri, Marinka Zitnik, Himabindu Lakkaraju
Advances in Neural Information Processing Systems (NeurIPS), 2022.
pdf -
Data Poisoning Attacks on Off-Policy Evaluation Methods
Elita Lobo, Harvineet Singh, Marek Petrik, Cynthia Rudin, Himabindu Lakkaraju
Conference on Uncertainty in Artificial Intelligence (UAI), 2022.
Oral Presentation [Top 5%]
pdf -
Exploring Counterfactual Explanations Through the Lens of Adversarial Examples: A Theoretical and Empirical Analysis.
Martin Pawelczyk, Chirag Agarwal, Shalmali Joshi, Sohini Upadhyay, Himabindu Lakkaraju
International Conference on Artificial Intelligence and Statistics (AISTATS), 2022.
pdf -
Probing GNN Explainers: A Rigorous Theoretical and Empirical Analysis of GNN Explanation Methods.
Chirag Agarwal, Marinka Zitnik, Himabindu Lakkaraju
International Conference on Artificial Intelligence and Statistics (AISTATS), 2022.
pdf -
Fairness via Explanation Quality: Evaluating Disparities in the Quality of Post hoc Explanations.
Jessica Dai, Sohini Upadhyay, Ulrich Aivodji, Stephen Bach, Himabindu Lakkaraju
AAAI/ACM Conference on AI, Society, and Ethics (AIES), 2022.
pdf -
Towards Robust Off-Policy Evaluation via Human Inputs.
Harvineet Singh, Shalmali Joshi, Finale Doshi-Velez, Himabindu Lakkaraju
AAAI/ACM Conference on AI, Society, and Ethics (AIES), 2022.
pdf -
A Human-Centric Take on Model Monitoring.
Murtuza N Shergadwala, Himabindu Lakkaraju, Krishnaram Kenthapadi
AAAI Conference on Human Computation and Crowdsourcing (HCOMP), 2022.
pdf -
Towards the Unification and Robustness of Post hoc Explanation Methods.
Sushant Agarwal, Shahin Jabbari, Chirag Agarwal*, Sohini Upadhyay*, Steven Wu, Himabindu Lakkaraju
Symposium on Foundations of Responsible Computing (FORC), 2022.
pdf -
Towards Robust and Reliable Algorithmic Recourse.
Sohini Upadhyay*, Shalmali Joshi*, Himabindu Lakkaraju
Advances in Neural Information Processing Systems (NeurIPS), 2021.
Best Paper Runner Up, ICML Workshop on Algorithmic Recourse, 2021.
pdf -
Reliable Post hoc Explanations: Modeling Uncertainty in Explainability.
Dylan Slack, Sophie Hilgard, Sameer Singh, Himabindu Lakkaraju
Advances in Neural Information Processing Systems (NeurIPS), 2021.
pdf -
Counterfactual Explanations Can Be Manipulated
Dylan Slack, Sophie Hilgard, Himabindu Lakkaraju, Sameer Singh
Advances in Neural Information Processing Systems (NeurIPS), 2021.
pdf -
Learning Models for Algorithmic Recourse
Alexis Ross, Himabindu Lakkaraju, Osbert Bastani
Advances in Neural Information Processing Systems (NeurIPS), 2021.
pdf -
Towards the Unification and Robustness of Perturbation and Gradient Based Explanations.
Sushant Agarwal, Shahin Jabbari, Chirag Agarwal*, Sohini Upadhyay*, Steven Wu, Himabindu Lakkaraju
International Conference on Machine Learning (ICML), 2021.
Spotlight Presentation
pdf -
Towards a Unified Framework for Fair and Stable Graph Representation Learning.
Chirag Agarwal, Himabindu Lakkaraju, Marinka Zitnik
Conference on Uncertainty in Artificial Intelligence (UAI), 2021.
pdf -
Fair influence maximization: A welfare optimization approach.
Aida Rahmattalabi, Shahin Jabbari, Himabindu Lakkaraju, Phebe Vayanos, Eric Rice, Milind Tambe
AAAI International Conference on Artificial Intelligence (AAAI), 2021.
pdf -
Does Fair Ranking Improve Minority Outcomes? Understanding the Interplay of Human and Algorithmic Biases in Online Hiring.
Tom Suhr, Sophie Hilgard, Himabindu Lakkaraju
AAAI/ACM Conference on Artificial Intelligence, Ethics, and Society (AIES), 2021.
pdf -
Beyond Individualized Recourse: Interpretable and Interactive Summaries of Actionable Recourses
Kaivalya Rawal and Himabindu Lakkaraju.
Advances in Neural Information Processing Systems (NeurIPS), 2020.
pdf -
Incorporating Interpretable Output Constraints in Bayesian Neural Networks
Wanqian Yang, Lars Lorch, Moritz Gaule, Himabindu Lakkaraju, Finale Doshi-Velez.
Advances in Neural Information Processing Systems (NeurIPS), 2020.
Spotlight Presentation [Top 3%]
pdf
-
Robust and Stable Black Box Explanations.
Himabindu Lakkaraju, Nino Arsov, Osbert Bastani.
International Conference on Machine Learning (ICML), 2020.
pdf -
Fooling LIME and SHAP: Adversarial Attacks on Post hoc Explanation Methods.
Dylan Slack, Sophie Hilgard, Emily Jia, Sameer Singh, Himabindu Lakkaraju.
AAAI/ACM Conference on Artificial Intelligence, Ethics, and Society (AIES), 2020.
Oral Presentation
pdf
Press: deeplearning.ai | Harvard Business Review -
"How do I fool you?": Manipulating User Trust via Misleading Black Box Explanations.
Himabindu Lakkaraju, Osbert Bastani.
AAAI/ACM Conference on Artificial Intelligence, Ethics, and Society (AIES), 2020.
Oral Presentation
pdf -
Faithful and Customizable Explanations of Black Box Models.
Himabindu Lakkaraju, Ece Kamar, Rich Carauna, Jure Leskovec.
AAAI/ACM Conference on Artificial Intelligence, Ethics, and Society (AIES), 2019.
Oral Presentation
pdf Human Decisions and Machine Predictions.
Jon Kleinberg, Himabindu Lakkaraju, Jure Leskovec, Jens Ludwig, Sendhil Mullainathan.
Quarterly Journal of Economics (QJE), 2018.
Featured in MIT Technology Review, Harvard Business Review, The New York Times,
and as Research Spotlight on National Bureau of Economics front page .
pdfThe Selective Labels Problem: Evaluating Algorithmic Predictions in the Presence of Unobservables.
Himabindu Lakkaraju, Jon Kleinberg, Jure Leskovec, Jens Ludwig, Sendhil Mullainathan.
ACM SIGKDD Conference on Knowledge Discovery and Data Mining (KDD), 2017.
Oral Presentation
pdfLearning Cost-Effective and Interpretable Treatment Regimes.
Himabindu Lakkaraju, Cynthia Rudin.
International Conference on Artificial Intelligence and Statistics (AISTATS), 2017.
INFORMS Data Mining Best Paper Award .
Invited Talk at INFORMS Annual Meeting .
pdfIdentifying Unknown Unknowns in the Open World: Representations and Policies for Guided Exploration.
Himabindu Lakkaraju, Ece Kamar, Rich Caruana, Eric Horvitz.
AAAI Conference on Artificial Intelligence (AAAI), 2017.
Featured in Bloomberg Technology .
pdfInterpretable and Explorable Approximations of Black Box Models.
Himabindu Lakkaraju, Ece Kamar, Rich Caruana, Jure Leskovec.
KDD Workshop on Fairness, Accountability, and Transparency in Machine Learning (FAT ML), 2017.
Invited Talk at INFORMS Annual Meeting .
pdfConfusions over Time: An Interpretable Bayesian Model to Characterize Trends in Decision Making.
Himabindu Lakkaraju, Jure Leskovec.
Advances in Neural Information Processing Systems (NIPS), 2016.
pdfInterpretable Decision Sets: A Joint Framework for Description and Prediction.
Himabindu Lakkaraju, Stephen H. Bach, Jure Leskovec.
ACM SIGKDD Conference on Knowledge Discovery and Data Mining (KDD), 2016.
Invited Talk at INFORMS Annual Meeting .
pdfMining Big Data to Extract Patterns and Predict Real-Life Outcomes.
Michal Kosinki, Yilun Wang, Himabindu Lakkaraju, Jure Leskovec.
Psychological Methods, 2016.
pdfLearning Cost-Effective and Interpretable Regimes for Treatment Recommendation.
Himabindu Lakkaraju, Cynthia Rudin.
NIPS Workshop on Interpretable Machine Learning in Complex Systems, 2016.
pdfLearning Cost-Effective and Interpretable Treatment Regimes for Judicial Bail Decisions.
Himabindu Lakkaraju, Cynthia Rudin.
NIPS Symposium on Machine Learning and the Law, 2016.
pdfDiscovering Unknown Unknowns of Predictive Models.
Himabindu Lakkaraju, Ece Kamar, Rich Caruana, Eric Horvitz.
NIPS Workshop on Reliable Machine Learning in the Wild, 2016.
pdfA Machine Learning Framework to Identify Students at Risk of Adverse Academic Outcomes.
Himabindu Lakkaraju, Everaldo Aguiar, Carl Shan, David Miller, Nasir Bhanpuri, Rayid Ghani.
ACM SIGKDD Conference on Knowledge Discovery and Data Mining (KDD), 2015.
Oral Presentation
pdfA Bayesian Framework for Modeling Human Evaluations.
Himabindu Lakkaraju, Jure Leskovec, Jon Kleinberg, Sendhil Mullainathan.
SIAM International Conference on Data Mining (SDM) , 2015.
Oral Presentation
pdfWho, When, and Why: A Machine Learning Approach to Prioritizing Students at Risk of not Graduating High School on Time.
Everaldo Aguiar, Himabindu Lakkaraju, Nasir Bhanpuri, David Miller, Ben Yuhas, Kecia Addison, Rayid Ghani.
Learning Analytics and Knowledge Conference (LAK), 2015.
pdfWhat's in a name ? Understanding the Interplay Between Titles, Content, and Communities in Social Media.
Himabindu Lakkaraju, Julian McAuley, Jure Leskovec.
International AAAI Conference on Weblogs and Social Media (ICWSM), 2013.
Oral Presentation
Featured in Time, Forbes, Phys.Org, Business Insider .
pdfDynamic Multi-Relational Chinese Restaurant Process for Analyzing Influences on Users in Social Media.
Himabindu Lakkaraju, Indrajit Bhattacharya, Chiranjib Bhattacharyya.
IEEE International Conference on Data Mining (ICDM), 2012.
Oral Presentation
pdfTEM: a novel perspective to modeling content on microblogs.
Himabindu Lakkaraju, Hyung-Il Ahn.
International World Wide Web Conference (WWW), short paper, 2012.
pdfExploiting Coherence for the Simultaneous Discovery of Latent Facets and associated Sentiments.
Himabindu Lakkaraju, Chiranjib Bhattacharyya, Indrajit Bhattacharya, Srujana Merugu.
SIAM International Conference on Data Mining (SDM), 2011.
Best Paper Award.
pdfAttention prediction on social media brand pages.
Himabindu Lakkaraju, Jitendra Ajmera.
ACM Conference on Information and Knowledge Management (CIKM), 2011.
pdfSmart news feeds for social networks using scalable joint latent factor models.
Himabindu Lakkaraju, Angshu Rai, Srujana Merugu.
International World Wide Web Conference (WWW), short paper, 2011.
pdf
Patents
Extraction and Grouping of Feature Words.
Himabindu Lakkaraju, Chiranjib Bhattacharyya, Sunil Aravindam, Kaushik Nath.
US8484228
Enhancing knowledge bases using rich social media.
Jitendra Ajmera, Shantanu Ravindra Godbole, Himabindu Lakkaraju, Bernard Andrew Roden, Ashish Verma.
US20130224714
Advising
I am very fortunate to be working with the following core group of students, interns, postdocs, and research affiliates
- Shichang Zhang (Postdoc, Harvard University)
- Aounon Kumar (Postdoc, Harvard University)
- Martin Pawelczyk (Postdoc, Harvard University); Co-advised with Seth Neel
- Usha Bhalla (PhD Student, Harvard University)
- Dan Ley (PhD Student, Harvard University)
- Alex Oesterling (PhD Student, Harvard University); Co-advised with Flavio Calmon
- Paul Hamilton (PhD Student, Harvard University)
- Zidi Xiong (PhD Student, Harvard University)
- Jenny Wang (PhD Student, Harvard University)
- Aaron Li (Masters Student, Harvard University)
- Zhenting Qi (Masters Student, Harvard University)
- Yanchen Liu (Masters Student, Harvard University)
Alumni (Past Advisees, Close Collaborators, and Visitors):
- Jiaqi Ma (Postdoc, Harvard University --> Assistant Professor, UIUC)
- Chirag Agarwal (Postdoc, Harvard University --> Assistant Professor, University of Virginia)
- Suraj Srinivas (Postdoc, Harvard University --> Research Scientist, Robert Bosch)
- Dylan Slack (PhD Student, UC Irvine --> Research Scientist, Google DeepMind)
- Satyapriya Krishna (PhD Student, Harvard University --> Research Scientist, Amazon)
- Tessa Han (PhD Student, Harvard University --> Postdoc, Harvard Medical School)
- Sree Harsha Tanneru (Masters Student, Harvard University --> Research Engineer, Google DeepMind)
- Aditya Karan (Masters Student, Harvard University --> PhD Student, UIUC CS)
- Kaivalya Rawal (Masters Student, Harvard University --> Research Fellow, Oxford University)
- Alexis Ross (Undergraduate Student, Harvard University -- Winner of Hoopes Prize for Best Undergrad Thesis --> PhD Student, MIT EECS)
- Isha Puri (Undergraduate Student, Harvard University --> PhD Student, MIT EECS)
- Jessica Dai (Undergraduate Student, Brown University --> PhD Student, UC Berkeley EECS)
- Eshika Saxena (Undergraduate Student, Harvard University --> AI Research Engineer, Meta)
- Ethan Kim (Undergraduate Student, Harvard University --> Founding Engineer, VectorShift)
- Catherine Huang (Undergrad, Harvard University --> Quant Trader, IMC Trading)
- Charu Badrinath (Undergrad, Harvard University --> Engineer, Palantir Technologies)
- Christina Xiao (Undergrad, Harvard University --> Engineer, Bloomberg)
- Sophie Hilgard (PhD Student, Harvard University --> Research Scientist, Twitter)
- Sushant Agarwal (Masters Student, University of Waterloo --> PhD Student, Northeastern University)
- Harvineet Singh (PhD Student, New York University; Research Intern, Harvard University --> Postdoc UCSF/UC Berkeley)
- Umang Bhatt (PhD Student, University of Cambridge; Research Intern, Harvard University --> Faculty Fellow, New York University)
- Ruijiang Gao (PhD Student, University of Texas at Austin; Research Intern, Harvard University --> Assistant Professor, UT Dallas)
- Elita Lobo (PhD Student, UMass Amherst; Research Intern, Harvard University)
- Anna Meyer (PhD Student, University of Wisconsin; Research Intern, Harvard University)
- Vishwali Mhasawade (PhD Student, New York University; Research Intern, Harvard University)
- Nick Kroeger (PhD Student, University of Florida; Research Intern, Harvard University)
- Chhavi Yadav (PhD Student, UC San Diego; Research Intern, Harvard University)
- Tom Suhr (MS Student, TU Berlin; Research Fellow, Harvard University --> PhD Student, Max Planck Institute)
- Davor Ljubenkov (Fullbright Scholar; Research Fellow, Harvard University)
Teaching
Introduction to Data Science and Machine Learning
Instructor
Harvard University, Fall 2020 - 2023.
Explainable AI: From Simple Predictors to Complex Generative Models
Instructor
Harvard University, Fall 2019, Spring 2021, Spring 2023.
Introduction to Data Science
Guest Lecture
Stanford Law School, 2016.
Probability with Mathemagic
Co-Instructor
Stanford Splash Initiative for High School Students, 2016.
Mining Massive Datasets Course
Teaching Assistant
Stanford Computer Science, 2016.
Submodular Optimization
Guest Lecture
Mining Massive Datasets Course, Stanford, 2016.
Social and Information Network Analysis Course
Head Teaching Assistant
Stanford Computer Science, 2014.
Machine Learning Course
Teaching Assistant
Indian Institute of Science, 2010.
English and Mathematics
Tutor
UNICEF's Teach India Initiative, 2008 - 2010.